The Aggregate 🔬 January 13th, 2020
Hello! I am Lars E. Schonander, a writer for MediaFile and a blogger on international affairs, tech, and general wonkery. Happy Monday! Here is my weekly newsletter with a weekly analysis with interesting data, along with links related to things I found particularly interesting that week. Any Questions? Send me a message or just respond to this email!
The Weekly Data:
Two weeks ago, I looked at a week’s work of data from the Metropolitan Transport Authority Transport website. The data starts in 2010, but a stable data format only begins in the end of 2014. To begin to download this data, I scraped the Turnstile data website to have the following format of title, date, with title containing the text file, and the date being a date in R that can later be filtered to include or exclude given time periods.
I spent at least 5 hours downloading the data, as initially I was going to download it into a SQLite database, but as SQLite does not support lag functions, I instead switched to a PostgreSQL database to store the dataset in.
Next, after all the data was downloaded, I had 45,000,000 rows of data essentially, and it crashed RStudio every time I tried to load it. R via DBPLYR lets one write DPLYR as SQL in a database, and as a function can print out the equivalent SQL function. With this SQL, I cleaned up via the usage of WITH statements to optimize the SQL to do any filtering and querying work in SQL, instead of R. I then use that query to create a table which was the one I was going to look at in practice. Despite the filtering, this new table ended up being over 500,000 rows large.
CREATE TABLE summary AS(
WITH lag_table AS
(SELECT
"STATION",
"DATE",
"ENTRIES" - LAG("ENTRIES", 1, NULL) OVER () AS "Entry Dif",
"EXITS" - LAG("EXITS", 1, NULL) OVER () AS "Exit Dif"
FROM "Sample"),
SUMMARY AS
(
SELECT "STATION", "DATE", SUM ("Entry Dif") as "TOTAL ENTRY", SUM ("Exit Dif") as "TOTAL EXIT"
FROM lag_table
GROUP BY "STATION", "DATE"
)
SELECT "STATION", "DATE", "TOTAL ENTRY", "TOTAL EXIT", "TOTAL ENTRY" + "TOTAL EXIT" as "TOTAL USAGE" FROM SUMMARYWHERE ("TOTAL ENTRY" + "TOTAL EXIT" > 0.0 AND "TOTAL ENTRY" + "TOTAL EXIT" < 100000.0)
);;
This ended up being the version of the data I was working with.
STATION", "DATE", "TOTAL ENTRY", "TOTAL EXIT", "TOTAL USAGE"
"Day","Month","Year"
With Day, Month, and Year being based off the DATE variable via the usage of several Lubridate functions.
System Wide View
Now that the data is actually ready, there are several analysis that are possible. For example, here is a graphic set up to look at the total usage of the Subway System per month for each year the data was collected.
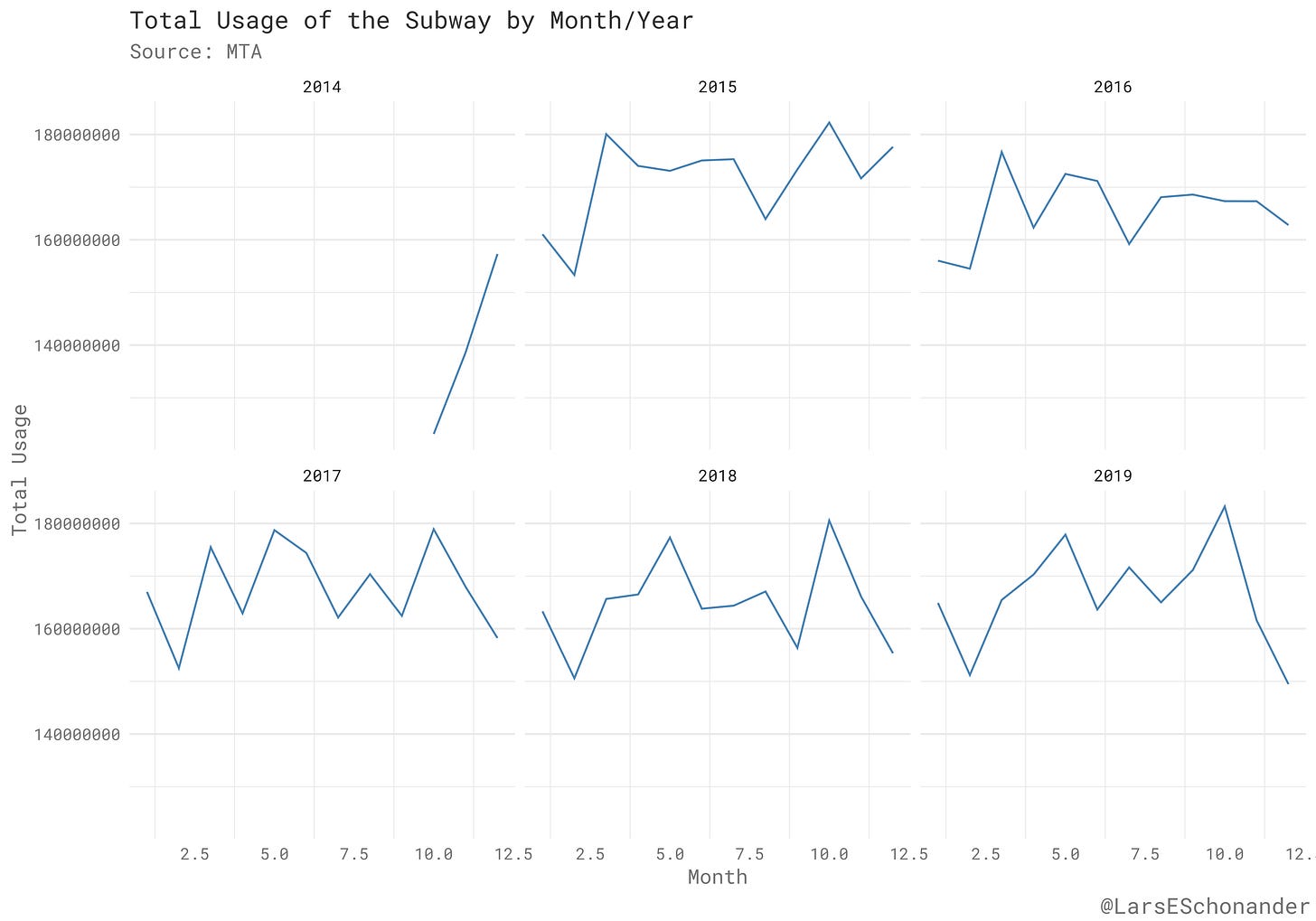
As one would expect the least busy months are when the seasons are extreme. In order, the top five least busy months is February, January, September, July, and August. The winter and summer months for lack of activity make sense as the New York city subway can be uncomfortable (at least when waiting for a train), in those periods. October, November, and December are the most busy months of the New York city subway system in practice, when aggregating by only year, and not by each month.
The following image shows that despite when looking at the data that year to year usage is constant, this plays out with some months having much lower levels of subway usage then other months.
Down to a Station
One can also look at the activity of a individual subway station as well. For example, here is the activity of Wall Street Station on the Green Line from the end of 2014 to the end of 2019.
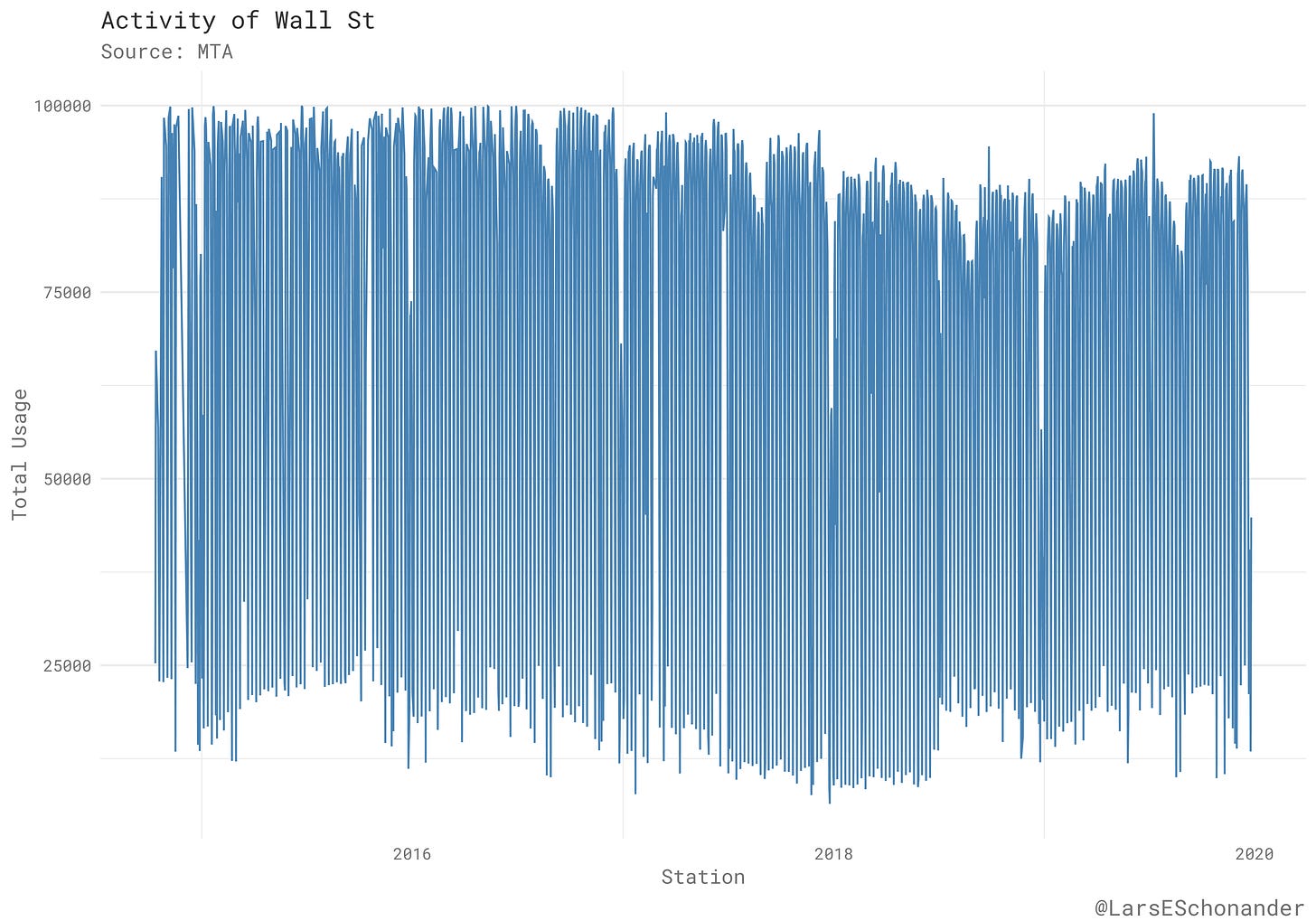
The most busy years for the station were in 2017 and 2018. One can create this type of chart for any station in the subway system that has a name.
Other Useful Measures
What the original dataset lacks is a notification when a day in the dataset is a major holiday or not. Using some approximations for when the day of a holiday falls in a given week, one can see how the usage of the subway is split between different events.
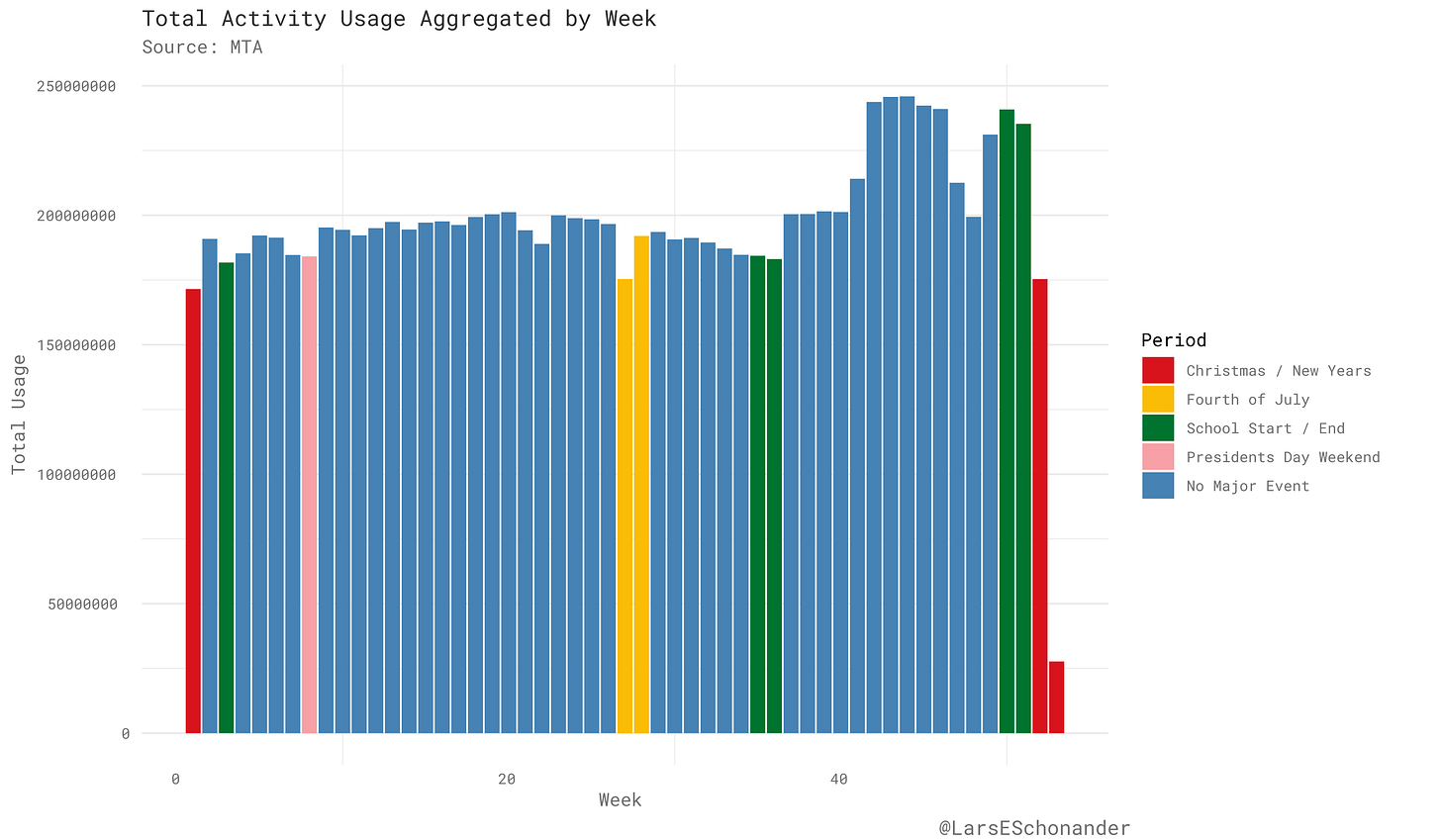
In general, more people use the subway at the end of year around October and November, then they do at the beginning on the year. Major holiday periods tend to be times when their is a drop in activity, like in the weeks before Thanksgiving or the Fourth of July. The end of November/Start of December however, is a period major activity within the subway system.
Now, some links…
Robert Trivers: Trivers on Epstein
I first met Jeffrey Epstein in 2004 when he threw a party in my honor at the Institute for Theoretical Biology, which he had set up at Harvard to the tune of a $6,000,000 grant. I was on sabbatical and Martin Nowak who ran the Institute granted me space (Photo 1).
Second time I met Jeffrey was at his home in NYC. He invited me to a party in honor of a very creative physicist at Princeton’s Institute of Advanced Studies, Freeman Dyson. A most agreeable party, mostly men but a very agreeable woman also, perhaps ten years younger, attractive, warm and friendly. Her name was Ghislane Maxwell. At some point we exchanged phone numbers but I do not remember any follow-up.
Dan Wang: 2019 Letter
Following the twists and turns of the trade war meant that I had less time for personal writing this year, so this letter is the only piece I’ll publish. I’m disappointed not to write more here, but on the other hand it might allow us to identify, for academic purposes, my lack of personal output to be the smallest and most trivial casualty of the trade war.
This year I want to discuss mostly science and technology. First, some thoughts on China’s technology efforts. Then I’ll present a few reflections on science fiction, with a focus on Philip K. Dick and Liu Cixin. Next I’ll discuss books I read on American industrial history. I save personal reflections for the end.
Adam Shatz (LRB): Trump Declares War
Qasem Soleimani – a major-general in Iran’s Revolutionary Guard and the leader of its Quds Force, a unit responsible for external and clandestine operations – was once described by Ayatollah Ali Khamenei, the Islamic Republic’s supreme leader, as ‘a living martyr of the revolution’. The living martyr is now a dead martyr, killed in an American airstrike along with five other people at Baghdad airport. Khamenei, who promoted Soleimani posthumously to lieutenant-general, has tweeted that ‘harsh vengeance’ is forthcoming; Hassan Nasrallah, the secretary-general of Lebanese Hizbullah, has declared that it is the ‘responsibility and task of all resistance fighters worldwide’ to avenge their deaths.
Serra Boranbayab Carmine Guerriero(Science Direct): A novel dataset on a culture of cooperation and inclusive political institutions in 90 European historical regions observed between 1000 and 1600
A culture of cooperation, which is the implicit reward from cooperating in any prisoner's dilemma and investment types of activity, and inclusive political institutions, which enable the citizenry to better select public-spirited representatives and check their decisions, are key for economic development. To foster research on the determinants and impact of these institutions, we illustrate a novel data set employed in [1] and [4] and gathering a measure of the activity of the Cistercians and the Franciscans, which is a proxy for the citizens' culture, and a constraints on the elite's decision-making power score, which is a proxy for the inclusiveness of political institutions, for a panel of 90 European historical regions spanning the 1000–1600 period.
Radu Soricut and Zhenzhong Lan (Google AI Blog): ALBERT: A Lite BERT for Self-Supervised Learning of Language Representations
Ever since the advent of BERT a year ago, natural language research has embraced a new paradigm, leveraging large amounts of existing text to pretrain a model’s parameters using self-supervision, with no data annotation required. So, rather than needing to train a machine-learning model for natural language processing (NLP) from scratch, one can start from a model primed with knowledge of a language. But, in order to improve upon this new approach to NLP, one must develop an understanding of what, exactly, is contributing to language-understanding performance — the network’s height (i.e., number of layers), its width (size of the hidden layer representations), the learning criteria for self-supervision, or something else entirely?
What I’m Reading
Trade From Space: Shipping Networks and The Global Implications of Local Shocks
A interesting little research paper that combines econometrics with the usage of alternative data sources to look at international trade across the world.
What I’m Working On
Writing a piece of the troubles of “move fast and break things”[1] when it comes to industrial policy and innovation, in reference to the idea of “progress studies” which has been bandied around for the past few months. Specifically, I plan to write about the rise of the first credit card networks. I hope to get it out by next week.
[1] Personally I am in agreement more often then not with the idea, but sometimes it’s worth being cautious.
Thanks!
Thanks for taking the time to read this, I will be back next Monday. In the meantime, you can follow me on Twitter or reach out via email.