Hello! I am Lars E. Schonander, a writer for MediaFile and a blogger on international affairs, tech, and general wonkery. Happy Monday! Here is my weekly newsletter with a weekly analysis with interesting data, along with links related to things I found particularly interesting that week. Any Questions? Send me a message or just respond to this email!
The Weekly Data:
Expanding on the dataset of 49085 BuzzFeed news articles, I decided to do more complicated types of textual analysis to find more interesting stories in the data, along with learn techniques that might be presented in The Pudding article I want to pitch.
To start, I began by doing Topic Modeling by performing a Latent Dirichlet Allocation to create a topic model of the dataset I been working with.
Below is a topic model I created of the dataset, where I specified 10 different topics to be created. Honestly specify the amount of topics is more art than a science, and requires some fiddling to get correct.
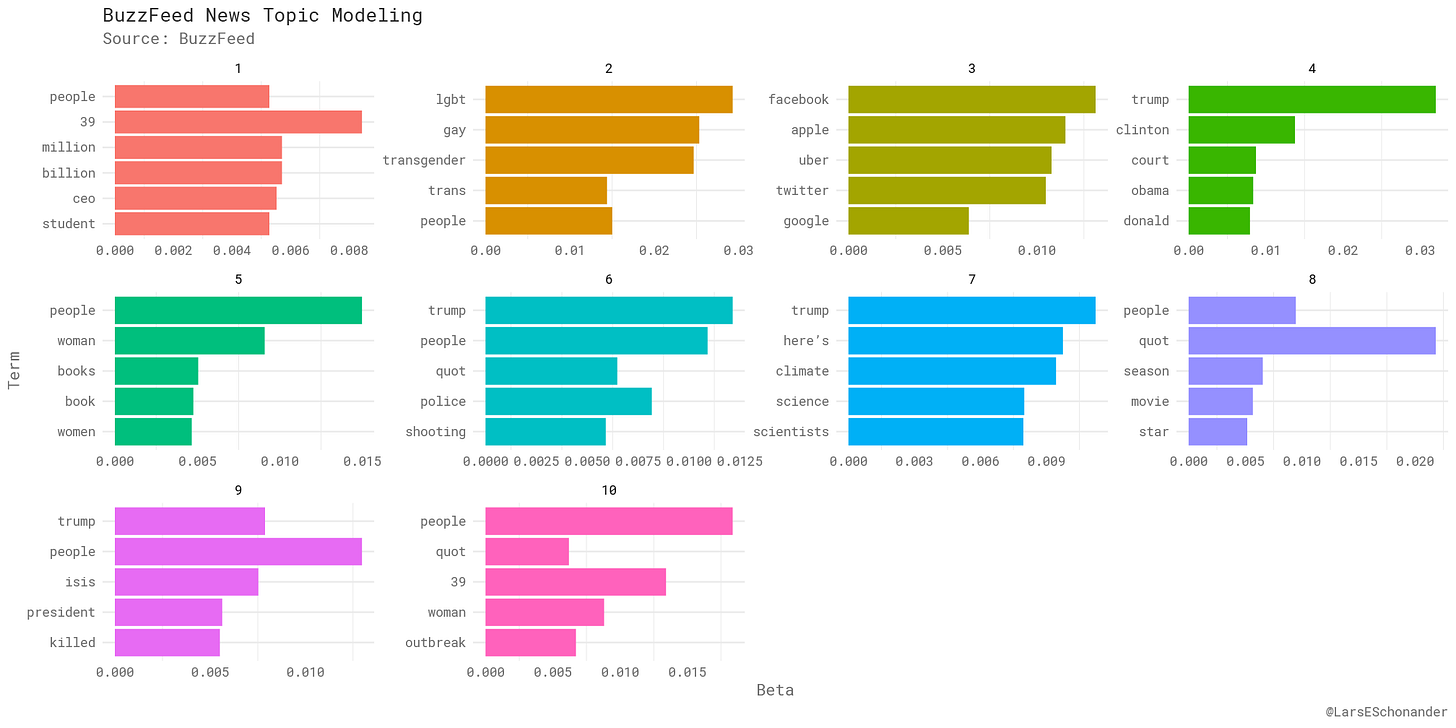
As seen above, quite a few of these topics have to do with Donald Trump in some capacity, considering many of the more recent articles have to deal with them. However, there is a unique topic for LGTBQ issues, along with looks to be student debt along with technology companies. There is also a topic for what seems to be movies and book reviews as well, which must mean the titles for those articles must be distinct in comparison to the other BuzzFeed news titles.
The next analysis is a small part of some word embedding I did on the dataset.
What was done in this case was a form Principal Component Analysis, a method of dimensionality reduction backed by quite a bit of Linear Algebra, to find the principle components of a dataset. While only a small section of word vectors, what this plot shows is that the relations have to do with various stories, such as Republican and Texas. Syria for example, is also near investigation.
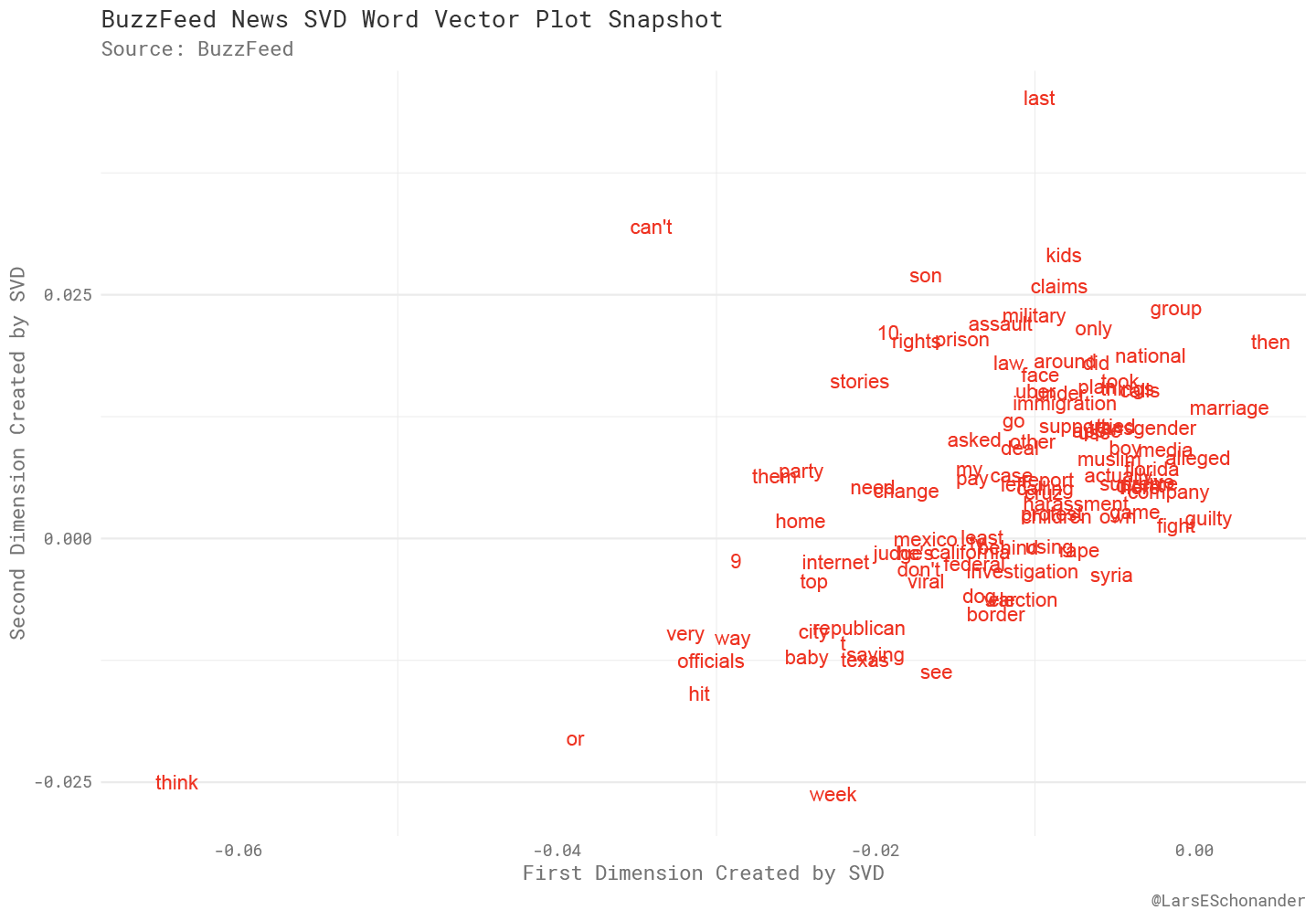
Word vectors can combined with a way to search words can also be used to do a cross reference of finding what word vector is similar to another word vector.
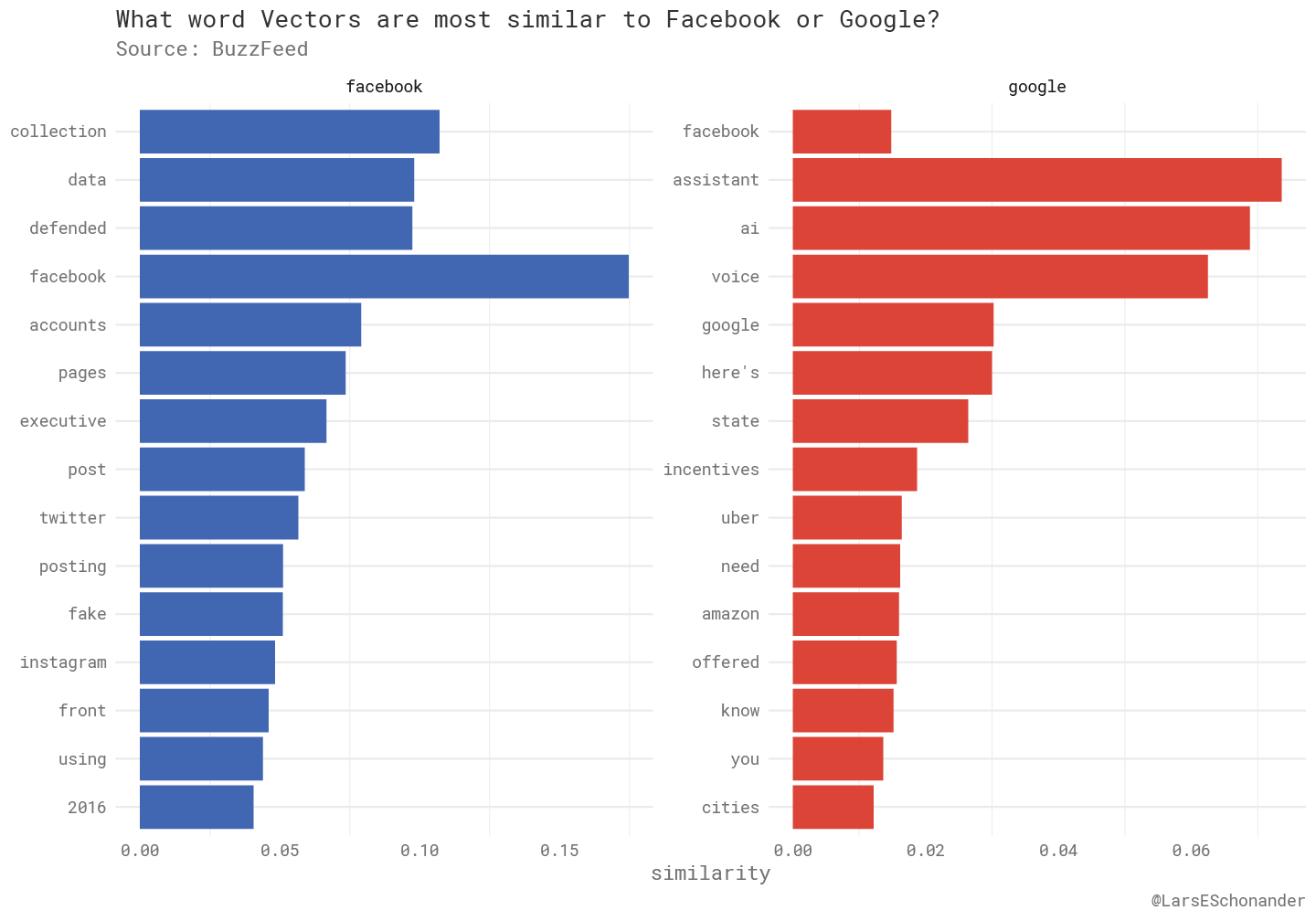
It is interesting to see how different the embeddings are for Facebook and Google. Google’s embeddings also include other tech companies, like Uber or Amazon, while Facebook’s word embeddings have to do with related issues, such as Instagram or the 2016 American presidential elections.
Now, some links…
Kevin Rogan (CityLab): The 3 Pictures That Explain Everything About Smart Cities
Suddenly, the world is awash in smart cities. Google is about to build one in Toronto. Hudson Yards in New York City is one, kind of. China and India have both declared national missions to construct or retrofit dozens of smart city projects all at once. And global tech giants and startups are racing to offer customers urban dashboards, “smart’’ systems, and new-age transit networks to make life happier and more efficient.
But no one seems able to agree on what a smart city actually is. Are they “the intersection of digital technology, disruptive innovation and urban environments”? Or “a place where traditional networks and services are made more efficient with the use of digital and telecommunication technologies”? To some, they’re a revolutionary “blueprint for the 21st-century urban neighborhood” that merges the “physical and digital realms.”
Matt Stoller & Lucas Kunce (The American Conservative): America’s Monopoly Crisis Hits the Military
This story of lost American leadership and production is not unique. In fact, the destruction of America’s once vibrant military and commercial industrial capacity in many sectors has become the single biggest unacknowledged threat to our national security. Because of public policies focused on finance instead of production, the United States increasingly cannot produce or maintain vital systems upon which our economy, our military, and our allies rely. Huawei is just a particularly prominent example.
Chris Moody (Stitch Fix): Stop Using Word2Vec
When I started playing with word2vec four years ago I needed (and luckily had) tons of supercomputer time. But because of advances in our understanding of word2vec, computing word vectors now takes fifteen minutes on a single run-of-the-mill computer with standard numerical libraries1. Word vectors are awesome but you don’t need a neural network – and definitely don’t need deep learning – to find them2. So if you’re using word vectors and aren’t gunning for state of the art or a paper publication then stop using word2vec.
What I’m Reading
Still going through the Algorithm Design Manual, taking notes chapter by chapter.
What I’m Working On
A reader informed me that they were wondering how the next democratic debate schedule is going to be set up. For the debates at the end of July, no one is going to be dropped out. However, for the debates after that, the criteria is going to become stricter. It will become 130,000 individual donors, along with a average polling number of 2%.
Thanks!
Thanks for taking the time to read this, I will be back next Monday. In the meantime, you can follow me on Twitter or reach out via email.